Human Factors and Simulation
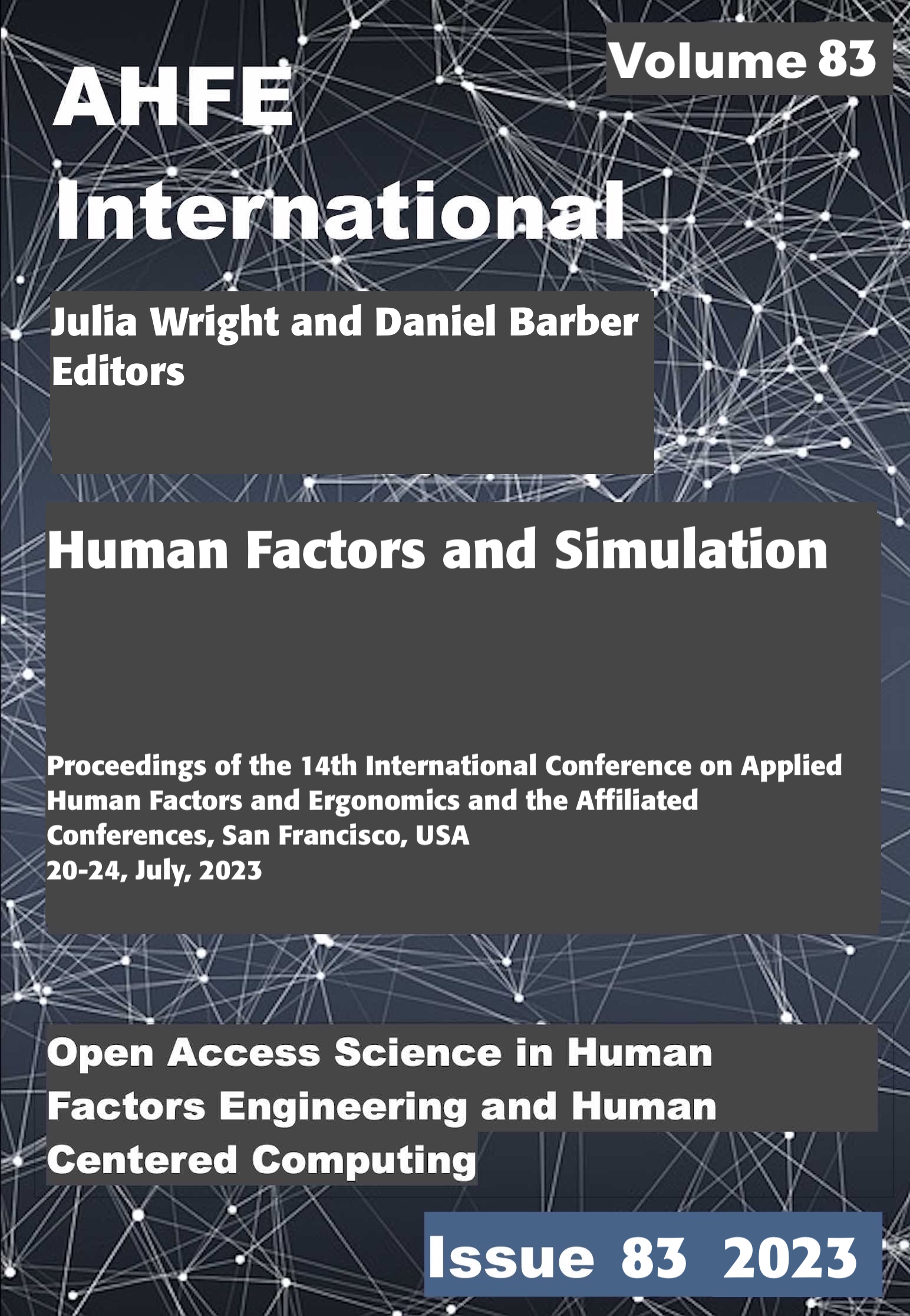
Editors: Julia Wright, Daniel Barber
Topics: Simulation and Modelling
Publication Date: 2023
ISBN: 978-1-958651-59-9
DOI: 10.54941/ahfe1003987
Articles
Team Plan Recognition: A Review of the State of the Art
There is an increasing need to develop artificial intelligence systems that assist groups of humans working on coordinated tasks. These systems must recognize and understand the plans and relationships between actions for a team of humans working toward a common objective. This article reviews the literature on team plan recognition and surveys the most recent logic-based approaches for implementing it. First, we provide some background knowledge, including a general definition of plan recognition in a team setting and a discussion of implementation challenges. Next, we explain our reasoning for focusing on logic-based methods. Finally, we survey recent approaches from two primary classes of logic-based methods (plan library-based and domain theory-based). We aim to bring more attention to this sparse but vital topic and inspire new directions for implementing team plan recognition.
Loren Rieffer-Champlin
Open Access
Article
Conference Proceedings
Beyond the tool vs. teammate debate: Exploring the sidekick metaphor in human-AI dyads
From symbiosis to copilot, a wide range of metaphors have been employed to characterize cooperative and collaborative relationships between human and non-human agents (be they software, robots, algorithms, or automated agents of any kind) in support of designing such advanced technologies. Recently, the emergence and rapid commoditization of artificial intelligence (AI) and machine learning (ML) algorithms have driven a highly bimodal debate on what metaphor is best to account for AI’s and ML’s new capabilities, particularly when those closely mimic humans’: Is AI a tool or a teammate for humans using the technology? This debate, however, occludes critical elements necessary to practitioners in the fields of human system design. To move past the “tool vs. teammate debate,” we propose an orthogonal metaphor, that of a sidekick, inspired by popular and literary culture, which can both accomplish and facilitate work (i.e., they do, and they help do). The sidekick metaphor was applied to a variety of efforts where it yielded novel design considerations which would have otherwise been unattainable by previous approaches. In this contribution, we report on the debate, describe the sidekick metaphor, and exemplify its application to real-world use cases, in domains such as intelligence analysis, aircraft maintenance, and missile defense.
Sylvain Bruni, Mary Freiman, Kenyon Riddle
Open Access
Article
Conference Proceedings
Measurement and Manipulation in Human-Agent Teams: A Review
In this era of the Fourth Industrial Revolution, increasingly autonomous and intelligent artificial agents become more integrated into our daily lives. As such, these agents are capable of conducting independent tasks within a teaming setting, while also becoming more socially invested in the team space. While ample human-teaming theories help understand, explain, and predict the outcome of team endeavors, such theories are not yet existent for human-agent teaming. Furthermore, the development and evaluations of agents are constantly evolving. As a result, many developers utilize their own test plans and their own measures making it difficult to compare findings across agent developers. Many agent developers looking to capture human-team behaviors may not sufficiently understand the benefits of specific team processes and the challenges of measuring these constructs. Ineffective team scenarios and measures could lead to unrepresentative training datasets, prolonged agent development timelines, and less effective agent predictions. With the appropriate measures and conditions, an agent would be able to determine deficits in team processes early enough to intervene during performance. This paper is a step in the direction toward the formulation of a theory of human-agent teaming, wherein we conducted a literature review of team processes that are measurable in order to predict team performance and outcomes. The frameworks presented leverage multiple teaming frameworks such as Marks et al.’s (2001) team process model, the IMOI model (Ilgen, 20005), Salas et al.’s big five model (2005) as well as more modern frameworks on human agent teaming such as Carter-Browne et al. (2021). Specific constructs and measures within the “input” and “process” stages of these models were pulled and then searched within the team’s literature to find specific measurements of team processes. However, the measures are only half of the requirement for an effective team-testing scenario. Teams that are given unlimited amount of time should all complete a task, but only the most effective coordinative and communicative teams can do so in a time efficient manner. As a result, we also identified experimental manipulations that have shown to cause effects in team processes. This paper aims to present the measurement and manipulation frameworks developed under a DARPA effort along with the benefits and costs associated with each measurement and manipulation category.
Maartje Hidalgo, Summer Rebensky, Daniel Nguyen, Myke Cohen, Lauren Temple, Brent Fegley
Open Access
Article
Conference Proceedings
Measuring Trust in a Simulated Human Agent Team Task
Due to improvements in agent capabilities through technological advancements, the prevalence of human-agent teams (HATs) are expanding into more dynamic and complex environments. Prior research suggests that human trust in agents plays a pivotal role in the team’s success and mission effectiveness (Yu et al., 2019; Kohn et al., 2020). Therefore, understanding and being able to accurately measure trust in HATs is critical. The literature presents numerous approaches to capture and measure trust in HATs, including behavioral indicators, self-report survey items, and physiological measures to capture and quantify trust. However, deciding when and which measures to use can be an overwhelming and tedious process. To combat this issue, we previously developed a theoretical framework to guide researchers in what measures to use and when to use them in a HAT context (Ficke et al., 2022). More specifically, we evaluated common measures of trust in HATs according to eight criteria and demonstrated the utility of different types of measures in various scenarios according to how dynamic trust was expected to be and how often teammates interacted with one another. In the current work, we operationalize this framework in a simulation-based research setting. In particular, we developed a simulated search and rescue task paradigm in which a human teammate interacts with two subteams of autonomous agents to identify and respond to targets such as enemies, IEDs and trapped civilians. Using the Ficke et al. (2022) framework as a guide, we identified self-report, behavioral, and physiological measures to capture human trust in their autonomous agent counterparts, at the individual, subteam, and full team levels. Measures included single-item and multi-item self report surveys, chosen due to their accessibility and prevalence across research domains, as well as their simplistic ability to assess multifaceted constructs. These self-report measures will also be used to assess convergent validity of newly developed unobtrusive (i.e., behavioral, physiological) measures of trust. Further, using the six-step Rational Approach to Developing Systems-based Measures (RADSM) process, we cross-referenced theory on trust with available data from the paradigm to develop context-appropriate behavioral measures of trust. The RADSM process differs from traditional data-led approaches in that it is simultaneously a top-down (data-driven) and bottom-up (theory-driven) approach (Orvis, et al., 2013). Through this process, we identified a range of measures such as usage behaviors (to use or misuse an entity), monitoring behaviors, response time, and other context-specific actions to capture trust. We also incorporated tools to capture physiological responses, including electrocardiogram readings and galvanic skin responses. These measures will be utilized in a series of simulation-based experiments examining the effect of trust violation and repair strategies on trust as well as to evaluate the validity and reliability of the measurement framework. This paper will describe the methods used to identify, develop and/or implement these measures, the resulting measure implementation and how the resulting measurement toolbox maps onto the evaluation criteria (e.g., temporal resolution, diagnosticity), and guidance for implementation in other domains.
Cherrise Ficke, Arianna Addis, Daniel Nguyen, Kendall Carmody, Amanda Thayer, Jessica Wildman, Meredith Carroll
Open Access
Article
Conference Proceedings
The Role of Artificial Theory of Mind in Supporting Human-Agent Teaming Interactions
In this article we discuss the role of Artificial Theory of Mind (AToM) in supporting human-agent teaming interactions. Humans are able to interpret, understand, and predict another’s behavior by leveraging core socio-cognitive processes, generally referred to as Theory of Mind (ToM). A human’s ToM is critical to their ability to successfully interact with others, especially in the context of teaming. Considering the increasing role of AI in team cognition, there is an emerging need for agents capable of such complex socio-cognitive processes. We report findings from a large multi-organization research program, DARPA’s Artificial Social Intelligence Supporting Teams (ASIST), designed to study teamwork with socially intelligent artificial agents serving as team advisors. We focus on agent-to-human communications, including content, intended purpose, and, particularly, the use of AToM attributions in both covert agent explanations as rationale for giving a certain intervention, as well as the use of agents making overt ToM attributions of players in the intervention itself. The findings suggest that agent teammates are able to demonstrate AToM and that that interventions based upon these can influence team outcomes. We discuss the impact of the various types of ASI interventions and their effect on teams, and provide recommendations for future research on human-AI teaming.
Jessica Williams, Rhyse Bendell, Stephen Fiore, Florian Jentsch
Open Access
Article
Conference Proceedings
Evolution of Workload Demands of the Control Room with Plant Technology
The management and assessment of operator workload is a critical element of nuclear power plant (NPP) safety. Operators in the NPP main control room (MCR) often face workload that varies both quantitatively and qualitatively as immediate task demands change. Although workload is an intuitive construct, it is not easy to define and measure in practice. This paper reviews the conceptual and empirical challenges in workload assessment, discusses the evolution of workload in MCRs, and presents subjective workload data from recent U.S. Nuclear Regulatory Commission (NRC)’s Human Performance Test Facility (HPTF) studies. Designs for NPP control rooms will increasingly utilize new technology, ranging from digitization of I&C through automation of operator functions to eventual use of AI. Workload assessment can contribute to determining whether the technology reduces cognitive demands on operators or has detrimental effects, such as increasing the vulnerability to human errors. We advocate for a multidimensional workload assessment approach based on Multiple Resource Theory and workload assessment should be combined with measurements of other constructs such as situation awareness, teamwork, and trust to identify vulnerabilities to error in NPPs.
Jinchao Lin, Gerald Matthews, Jacquelyn Schreck, Kelly Dickerson, Niav Hughes
Open Access
Article
Conference Proceedings
Characterizing Complexity: A Multidimensional Approach to Digital Control Room Display Research
Complexity can be characterized at numerous levels; physical, perceptual, and cognitive features all influence the overall complexity of an informational display. The Human Performance Test Facility (HPTF) at the U.S. Nuclear Regulatory Commission (NRC) develops lightweight simulator studies to examine the workload induced by various control room-related tasks in expert and non-expert populations. During the initial development of the lightweight simulator, cognitive complexity was defined based on the number of elements in each control panel. While the number of items roughly maps onto information density, it is only one of several features contributing to display complexity. This study is a follow-up to the original complexity evaluation and includes an initial characterization of the perceptual complexity of a set of control panels in their original (i.e., unmodified) and modified (for cognitive complexity reduction) forms. To assess perceptual complexity, a 3-dimensional approach was developed. The control panel displays were assessed using common measures of physical complexity (e.g., edge congestion, clutter, symmetry), performance-based measures (reaction time and accuracy for target identification), and subjective impressions using a survey adapted from a similar FAA assessment of air traffic controller workstation display complexity. Overall, the results suggested that clutter and symmetry were associated with target identification performance; participants interacting with high symmetry-low clutter displays identified target controls faster than those interacting with low symmetry-high clutter displays. Survey results tended to follow the same pattern as the physical and performance-based results; however, these patterns were not statistically significant, likely due to the small sample size. These initial results are a promising indication that the physical and performance-based measures were valid for assessing display complexity and that they are sensitive to differences in complexity, even with smaller samples. The physical and performance-based measures may be good candidates for human factors validation of future system designs - they are quick and easy to administer while providing a holistic sense of display perceptual complexity. Like other types of surveys, surveys for display complexity often require large samples to detect meaningful differences between groups. System designers and other stakeholders may want to consider alternative strategies, such as physical system measurement and characterization using performance-based methods if the user base is small or designs are in the early stages of development, requiring quick answers and an iterative approach to evaluation.
Kelly Dickerson, John Grasso, Heather Watkins, Niav Hughes
Open Access
Article
Conference Proceedings
Evaluation of a Basic Principle SMR Simulator for Experimental Human Performance Research Studies
Simulator studies are important to understanding and collecting data on human performance, especially for first-of-a-kind technologies such as Small Modular Reactors (SMR) and/or in cases where the role of the human operator is expected to change, such as in multi-unit operations. But not all simulators are the same, and the level of complexity and fidelity of the simulator can significantly affect the possibilities for data collection. As a researcher, how can you evaluate whether the simulator you are using is suitable for the studies that you wish to run? In 2020, the researchers of the Halden Reactor Project (HRP) activity on operation of multiple small modular reactors had a unique opportunity to explore this question. A multi-unit basic principle integral pressurised water reactor (iPWR) simulator was installed in the FutureLab facility in Halden, Norway in early 2019, and a first, small study was conducted in late 2019 to test the simulator environment and study design. The simulator was provided to the HRP free of charge by the International Atomic Energy Agency (IAEA). It is important to note that the simulator was not designed for performance of research studies, but rather as an education tool to demonstrate the basic principles and concepts of SMR operation, and as such is limited in scope by design. The goal for the small study in 2019 was to determine whether the basic principle simulator could enable investigation of predefined topics relevant to SMR operations research, such as monitoring strategies, prioritization of taskwork and staffing requirements in multi-unit environments. The study involved two experienced former control room operators, who were tested individually in a series of scenarios of increasing complexity in a multi-unit control room setup, observed by experienced experimental researchers. Further studies with licensed control room operators were planned for 2020, but due to the COVID-19 pandemic these plans had to be postponed. Instead, the research team took the opportunity to reflect on the experience of the 2019 small study, to perform a more detailed analysis of the study results and to substantiate the feasibility of the test environment for future experimental data collection. The detailed analysis was performed in a workshop format with the research project team using a set of questions related to specific aspects of the iPWR 3-unit control room setup. The team used a “traffic light” rating system to evaluate each question, where red indicated that that the test environment item under discussion is not currently feasible and would require redesign and extensive changes in order to use it; yellow indicated that the item is not currently feasible and would require moderate changes, and green indicated that the item is currently feasible, and some minor changes could be required. This paper describes the evaluation process in more detail, including the criteria for assessing the usefulness of the basic principle simulator for conducting experimental human performance studies in the future, and the results of the evaluation. While the list of criteria identified during this workshop is not considered exhaustive, these results are still expected to be useful to other researchers considering experimental control room studies to determine the level of complexity and fidelity that can be reasonably achieved in a control room simulator.
Claire Blackett
Open Access
Article
Conference Proceedings
Behavioral indicators - an approach for assessing nuclear control room operators’ excessive cognitive workload?
Cognitive workload that deteriorates the control room team’s performance is a central topic for human-technology design and evaluation. However, while stated as an essential research topic, the literature provides few studies investigating the excessive cognitive workload of complex dynamic human-system work. Multiple techniques have been developed to sample workload. Still, they all struggle to determine the nature of excessive workload, capturing change but leaving the interpretation to the investigator. To advance the measurement of excessive cognitive workload of complex work, this paper proposes to investigate behavioral indicators. Behavioral-based methods differ from performance measures as they concentrate on the operator's behavior rather than the outcome of the actions. The information embedded in the operator’s behavior may not directly reflect the outcome of the task. The paper proposes indicator categories in terms of task prioritization, work practices and low-level behavior. The approach implies developing an understanding of how control room teams adapt to and manage task load and how operators are affected by high workload – for the identification of indicators, and for the development and validation of measures from these cognitive workload indicators. The paper presents an initial review of simulator studies identifying adaption such as down-prioritizing secondary tasks, reducing attention to global process overview, asking for or providing team support on task demand, reducing verification of work, and delayed response in communication. Furthermore, we briefly consider the technical and staffing requirements necessary to support these measures.
Per Øivind Braarud, Giovanni Pignoni
Open Access
Article
Conference Proceedings
Transfer of nuclear maintenance skills from virtual environments to reality - Toward a methodological guide
Nuclear maintenance operations require several types of cognitive and motor skills that can be trained in immersive environments. However, there is a lack of normalized methodological approaches to classify tasks and guide them for a potential transposition to immersive training. This paper proposes a methodological approach to classify nuclear maintenance tasks based on their complexity and the potential transfer of training obtainable from each type of immersion techniques and their related interactions.This proposed methodology provides a novel approach to compare various immersive technologies and interactions in a normalized way for a same industrial task.This paper aims at serving as a base for a methodological guide dedicated to the transposition of nuclear maintenance skills learned in immersive environments to real environment setups and proposes two future use cases based on this methodological approach.
Valérian Faure, Jean-Rémy Chardonnet, Daniel Mestre, Fabien Ferlay, Michaël Brochier, Laurent Joblot, Frédéric Merienne, Claude Andriot
Open Access
Article
Conference Proceedings
A Proposed Methodology to Assess Cognitive Overload using an Augmented Situation Awareness System
The US Army is tasked with providing the best tools to keep military personnel at peak performance. These tools can be found in many forms: small arms, protective clothing, armored vehicles, and communication devices, etc. However, understanding when a person is cognitively overloaded does not have such a tool. Cognitive overload is nothing new, yet it is not well understood. This paper discusses cognitive overload, why it is critical to military performance, past efforts, and focuses on a methodology to assess cognitive overload using a deployed augmented situation awareness (SA) system. We will employ a currently used SA system to assess cognitive overload through an additive process designed to identify when overload occurs and performance drops. Understanding when cognitive overload occurs is critical to Soldier survivability and offsetting it before it becomes a detriment is key. We will discuss our methodology assessing when cognitive overload occurs and potential mitigation strategies.
Debra Patton, Joshua Rubinstein
Open Access
Article
Conference Proceedings
Assessment of pilots' training efficacy as a safety barrier in the context of Enhanced Flight Vision Systems (EFVS)
Aviation and air travel have always been among the businesses at the forefront of technological advancement throughout history. Both the International Air Transportation Authority's (IATA) Technology Roadmap (IATA, 2019) and the European Aviation Safety Agency's (EASA) Artificial Intelligence (AI) roadmap (EASA, 2020) propose an outline and assessment of ongoing technological prospects that change the aviation environment with the implementation of AI from the initial phases. New technology increased the operational capabilities of airplanes in adverse weather. An enhanced flight vision system (EFVS) is a piece of aircraft equipment that captures and displays a scene image for the pilot, allowing for improved scene and object detection. Moreover, an EFVS is a device that enhances the pilot's vision to the point where it is superior to natural sight. An EFVS has a display for the pilot, which can be a head-mounted display or a head-up display, and image sensors such as a color camera, infrared camera, or radar. A combined vision system can be made by combining an EFVS with a synthetic vision system. A forward-looking infrared camera, also known as an enhanced vision system (EVS), and a Head-Up Display (HUD) are used to form the EFVS. Two aircraft types can house an EFVS: fixed-wing (airplane) and rotary-wing (helicopter).Several operators argue that the use of Enhanced Flight Vision Systems (EFVS) may be operated without the prior approval of the competent authority, assuming that the flight procedures, equipment, and pilot safety barriers are sufficiently robust. This research aims to test pilots' readiness levels with no or little exposure to EFVS to use such equipment (EASA, 2020). Moreover, the Purdue simulation center aims to validate this hypothesis. The Purdue human systems integration team is developing a test plan that could be easily incorporated into the systems engineering test plan to implement Artificial Intelligence (AI) in aviation training globally and evaluate the results. Based on guidelines from the International Air Transport Association (IATA), the Purdue University School of Aviation and Transportation Technology (SATT) professional flying program recognizes technical and nontechnical competencies. Furthermore, the Purdue Virtual Reality research roadmap is focused on the certification process (FAA, EASA), implementation of an AI training syllabus following a change management approach, and introduction of AI standardization principles in the global AI aviation ecosystem.
Dimitrios Ziakkas, Konstantinos Pechlivanis, Brian Dillman
Open Access
Article
Conference Proceedings
Participant Gaming Experience Predicts Mental Model Formation, Task Performance, and Teaming Behavior in Simulated Search and Rescue
Video gaming experience has been found to impact behavior and performance on experimental tasks, can influence cognitive processes, and may even transfer to tasking proficiency. The purpose of the investigations reported in this manuscript were jointly to examine the relationships between video game experience and mental model formation as well as experience and gameplay behaviors in the context of a game-based urban search and rescue mission. We hypothesized that differences in video game play experience would influence the formation of mental models, and that experience would also be associated with different behavioral tendencies during tasking. To test our hypotheses, we first conducted an investigation to evaluate the relationship between video game play experience and mental model formation given the context of a simulated urban search-and-rescue task (employing psychographic measures of gaming experience in addition to card sort mental model elicitation) and second drew on data collected under DARPA’s Artificial Social Intelligence Supporting Teams program (particularly, behavioral and performance metrics related to players' execution of mission critical actions and team supportive behaviors) to examine the influence of experience on individual and team tasking behaviors. Results of Study 1 support our hypothesis that greater video game experience was associated with more convergent mental models related to the game-based experimental task. Results of Study 2 indicate that participants with greater experience showed evidence of better overall performance and more strategic behavior. These findings suggest that video gaming experience impacts both the formation of task-related mental models as well as task performance and teaming behaviors. One critical takeaway from the results of these studies is that some aspects of generalized video game experience may transfer to novel task performance. Having found evidence of transfer in this context is particularly informative because the search-and-rescue task environment was essentially novel, and although it was based in Minecraft the task itself employed only the sandbox foundation and involved almost no features that appear in standard Minecraft survival or other modes. The video game experience measure, on the other hand, tapped general as well as Minecraft specific experience with respect to duration, frequency/intensity, and self-reported skill. These findings have implications for simulation based research methods, particularly with regards to identification and control of potential confounding variables, as well as the practical application of simulated training and testing. Further, we submit that gaming experience is emerging as a critical factor that may be used to profile participants across research, training, and operational domains for the purposes of predicting individual behavior and performance as well as to inform the formation and development of teams.
Rhyse Bendell, Jessica Williams, Stephen Fiore, Florian Jentsch
Open Access
Article
Conference Proceedings
Evaluating the Effectiveness of Mixed Reality as a Cybersickness Mitigation Strategy in Helicopter Flight Simulation
The advent of Virtual Reality (VR) in flight simulation promises to provide a cost-effective alternative for flight crew training compared to conventional flight simulation methods. However, it has been noted that the use of VR in flight simulation can lead to a greater incidence of cybersickness, which could jeopardize the effectiveness of flight training in VR. To optimally leverage the benefits that VR in flight simulation can bring, it is critical that this higher likelihood of experiencing cybersickness is countered. Even though a variety of theories for the causes for cybersickness in VR have been formulated, one of the most widely-accepted theories hinges on the principle that the sensory conflict between the visual sensory inputs from the virtual environment and the motion that is sensed by the vestibular system can result in cybersickness. Minimizing this sensory conflict can therefore be a strategy to mitigate cybersickness. The use of Mixed Reality (MR), in which the virtual environment is visually blended with the actual environment, could potentially be used for this strategy, based on the idea that it provides a visual reference of the actual environment that corresponds with the motion that is sensed, thereby reducing the sensory conflict and, correspondingly, cybersickness.The objective of this research is to investigate the effectiveness of MR, as an alternative for VR, for the mitigation of cybersickness in helicopter flight simulation. Since the idea of using MR as a cybersickness mitigation strategy is rooted in the idea of reducing the mismatch between visual and vestibular sensory inputs, the effectiveness of MR in combination with simulator motion is investigated as well. Arguably, MR could deteriorate immersion and reduce simulation fidelity, which may hamper the ability of the pilot to adequately fly in the virtual environment. Based on this premise, it is expected that a sweet spot exists where cybersickness is reduced, while fidelity remains sufficient to perform the flying task satisfactorily. In addition to evaluating the effectiveness for cybersickness mitigation, the impact of MR on pilot performance is also investigated.A human-in-the-loop experiment was performed that featured a total of four conditions, designed to assess the impact of both MR and motion on the cybersickness development and pilot performance. The experiment was performed in a simulated AgustaWestland AW139 helicopter on a Motion Systems’ PS-6TM-150 motion platform (6DoF), combined with a Varjo XR-3 visual device. In the experiment, Royal Netherlands Air Force helicopter pilots (n=4) were instructed to fly a series of maneuvers from the ADS-33 helicopter handling qualities guidelines. The Pirouette task in ADS-33 is the main focus for the results analysis because it is expected that near ground dynamic maneuvering affects cybersickness more severely compared to more stable and high altitude performed tasks.The cybersickness is evaluated by means of MIsery SCale (MISC) scores that were reported by the participants after the maneuver, and through a qualitative assessment triggered by VR comfort criteria. Pilot performance is assessed by examining the flight trajectories, helicopter inputs, and relevant helicopter outputs.The experiment campaign was completed in the last quarter of 2022, for which the results analysis is still ongoing. Preliminary results suggest that MR in the absence of simulator motion can have a beneficial effect on the development of cybersickness, as lower MISC scores were observed compared to the other conditions. However, the addition of motion with MR seems to have the opposite effect on cybersickness. Key in this result is that participants mentioned that being able to observe the simulator motion washout – the sensory-subliminal movement which allows the simulator to return to the neutral position – was an important sickening factor. The paper features a more in-depth analysis of the MISC scores and post-exposure sickness questionnaire results to further support the findings regarding the effect of MR and motion on cybersickness.While the analysis of the pilot performance results requires additional effort, current analysis of the flight trajectories and helicopter outputs suggests that MR may have a detrimental effect on pilot performance. The analysis of the pilots’ control input, however, still has to be completed. Next to an analysis of the flight trajectories and the helicopter outputs, the paper’s results also includes an analysis of the pilots’ control input – both in the time and frequency domains – to allow for a more detailed analysis on how the mitigating measures affect the individuals’ helicopter handling qualities and to which extent this influences the experienced cybersickness.
Boris Englebert, Laurie Marsman, Jur Crijnen
Open Access
Article
Conference Proceedings
Attention Military/Commercial Simulation Developers, Users, & Trainers: Visually-induced or Motion-induced Sickness is not Necessarily More Severe for Women
Extended reality (XR), head-mounted displays (HMD), simulators, and advanced vehicle/teleoperation display-control systems show promise for augmenting job skills training or aiding mission decision-making among aviators, astronauts, ship handlers, emergency responders, etc. Unfortunately, such systems require unnatural sensorimotor integration which often induces motion-sickness and/or visually-induced motion sickness (VIMS). NATO and other groups are studying who is most vulnerable, which will inform system design and training protocols. A common assertion is that most studies find women far more susceptible to motion sickness/VIMS, and a recent article called one type of virtual reality (VR) “sexist in its effects.” We reviewed how many studies support the notion that women are more susceptible. We amassed the largest known sample of relevant literature involving direct empirical or survey studies of potential sex difference among studies of motion sickness or VIMS. To date, 76 relevant studies have been identified, among which only 37 (48.7%) are consistent with the assertion that women are more susceptible than men. Such findings require researchers, developers, and trainers to refrain from concluding a sex difference exists presently, especially since many studies are not tightly controlled. Premature judgments could harm military/workforce readiness, career prospects of women, and dissemination of useful technologies.
Ben Lawson, Jeffrey Bolkhovsky
Open Access
Article
Conference Proceedings
Using Cognitive Models to Develop Digital Twin Synthetic Known User Persona
A recurring challenge in user testing is the need to obtain a record of user interactions that is large enough to reflect the different biases of a single user persona while accounting for temporal and financial constraints. One way to address this need is to use digital twins of user personas to represent the range of decisions that could be made by a persona. This paper presents a potential use of cognitive models of user personas from a single complete record of a persona to test the web-based decision support system, ALFRED the BUTLER. ALFRED the BUTLER is a digital cognitive assistant developed to generate recommended articles for users to review and evaluate relative to a priority information request (PIR).Interaction data for three different user personas for the ALFRED the BUTLER system were created: the Early Terminator, the Disuser, and the Feature Abuser. These three personas were named after the type of interaction they would have with the data and were designed to represent different types of human-automation user interactions as outlined by Parasuraman & Riley (1997). The research team operationalized the definitions of use, misuse, disuse, and abuse to fit the current context. Specifically, the Early Terminator represented misuse by no longer meaningfully interacting with the system once a search criterion was met whereas the Disuser represented disuse by never using a certain feature. The Feature Abuser represented abuse by excessively using a single feature when they should be using other features. Each member of the research team was assigned a user persona, given a briefing related to their persona, and instructed to rate 250 articles as either relevant (thumbs up), irrelevant (thumbs down), or neutral (ignore). Subsequently, a cognitive model of the task was built. Cognitive models rely on mechanisms that capture human cognitive processes such as memory, learning, and biases to make predictions about decisions that humans would be likely to make (Gonzalez & Lebiere, 2005). To construct the cognitive model, we relied on the Instance-Based Learning (IBL) Theory (Gonzalez et al., 2003), a cognitive theory of experience-based decision making. The data for each user’s previous actions were added to the model’s memory to make predictions about the next action the user would be likely to make (thumbs up, thumbs down, or ignore an article). The model was run 100 times for each persona, with the 250 articles presented in the same order as they were judged by the persona. The results indicate an overall model prediction accuracy of the persona’s decisions above 60%. Future work will focus on refining and improving the model's predictive accuracy The authors discuss future applications, one of which is using this type of cognitive modeling to help create synthetic datasets of persona behaviors for evaluation and training of machine learning algorithms.ReferencesGonzalez, C., & Lebiere, C. (2005). Instance-based cognitive models of decision-making.Gonzalez, C., Lerch, J. F., & Lebiere, C. (2003). Instance‐based learning in dynamic decision making. Cognitive Science, 27(4), 591-635.Parasuraman, R., & Riley, V. (1997). Humans and automation: Use, misuse, disuse, abuse. Human factors, 39(2), 230-253.
Audrey Reinert, Summer Rebensky, Maria Chaparro Osman, Baptiste Prebot, Cleotilde Gonzalez, Don Morrison, Valarie Yerdon, Daniel Nguyen
Open Access
Article
Conference Proceedings
The hybrid analysis as a disseminator in the field of motion economics studies through machine learning methods and rule-based knowledge
Manufacturing companies are increasingly confronted with the challenges of market globalisation, a shortening of product life cycles and a growing diversity of variants. New and flexible approaches to optimizing production processes and their planning ability are therefore needed to secure competitiveness in a sustainable way. Manual assembly in particular is a cost factor in the manufacturing industry and takes up a high proportion of the total production time. In addition to the efficient design of assembly processes, the ergonomic assessment and optimisation of work systems to avoid health hazards is also becoming increasingly important, also in consideration of demographic change. Currently, high personnel costs for the analysis of the workplace as well as special technical requirements for the employees in industrial engineering are identified as problematic. Especially for small and medium-sized companies with limited capacities in planning and existing competence levels of the employees, this aspect represents a hurdle that should not be underestimated. The following paper discusses the hypothesis that a combined approach of machine learning and rule-based knowledge as a hybrid analysis is suitable for transferring motion data captured by motion capturing into rule-conforming analyses in a semi-automated way. For this purpose, the new process building block system MTM-Human Work Design is used, which documents the required influencing factors chronologically and makes them variably evaluable in order to create time measurements and ergonomic execution analyses.
Steffen Jansing, Roman Moehle, Barbara Brockmann, Jochen Deuse
Open Access
Article
Conference Proceedings
User Need Assessment Using Simulator Feature Framework
Full-scope nuclear control room simulators were developed to address operator skill deficits associated with several high-profile accidents occurring in the 1980s. Full-scope simulators are increasingly used to support plant modernization and advanced reactor research and development. New digital control room designs use full-scope simulators to develop and evaluate new concept of operations to support regulator required Human Factors Engineering Program Review Model (HFEPRM) activities. Modern simulator designs require more diverse and robust capabilities to serve the diverse needs of multiple user groups including researchers and educators. A common framework for evaluating features to support training, research, and education is critical to ensure future simulators enable research to support immediate and future plant modernization and advanced reactor deployment needs. An initial framework comprised of eight feature categories was developed by reviewing published simulator-based research and analyzing simulator features against research objectives and results (Gideon and Ulrich, 2022). A survey was administered to simulator users to evaluate the suitability of eight critical capabilities of a modified version of the framework to characterize and differentiate simulators across training, research, and education uses (n = 21). The results demonstrate the framework's effectiveness as a baseline for assessing the functionalities of simulators in line with their specific needs. Future work aims to validate the framework within a regulatory HFEPRM process to demonstrate its use as a tool to identify missing capabilities of existing simulators or to specify requirements for new simulators.
Olugbenga Gideon, Thomas Ulrich
Open Access
Article
Conference Proceedings
A Cognitive Model for Guiding Automation
A variety of systems and exist for managing human-machine team throughput and effectiveness. One example is autonomous managers (AMs), software that dynamically reallocates tasks to individual members of a team based on their workload and performance. Cognitive models can inform these technologies by projecting performance into the future and enabling “what-if” analyses. For example, would removing a task from an individual whose current performance is low cause them to improve? Conversely, can a team member who is currently performing well handle even more work without dropping performance? In the present study, we develop and validate a cognitive model built in the Adaptive Control of Thought – Rational (ACT-R) cognitive architecture in a novel empirical paradigm: The Intelligence, Surveillance, and Reconnaissance Multi-attribute Task Battery (ISR-MATB). In this task, participants engage in a mock ISR task in which they must integrate information from several subtasks to arrive at a decision about a situation. These tasks include searching visual displays, listening for audio chatter, making decisions based on multiple cues, and remaining vigilant for signals. The tasks are based upon analogous laboratory psychology tasks to improve empirical rigor. Eight participants completed the task under two 30-minute conditions: easy and difficult. The difficult task required searching more complex stimuli in the audio and visual domain than in the easy condition. In addition, subjective workload ratings (NASA-TLX) were collected. We describe the preliminary behavioral and self-report results, as well as the ACT-R model’s fit to the behavioral data. Further, we describe a new method for workload visualization and task decomposition using model-based analyses.
Christopher Stevens, Christopher Fisher, Mary Frame
Open Access
Article
Conference Proceedings
Developing Confidence in Machine Learning Results
As the field of deep learning has emerged in recent years, the amount of knowledge and expertise that data scientists are expected to absorb and maintain has correspondingly increased. One of the challenges experienced by data scientists working with deep learning models is developing confidence in the accuracy of their approach and the resulting findings. In this study, we conducted semi-structured interviews with data scientists at a national laboratory to understand the processes that data scientists use when attempting to develop their models and the ways that they gain confidence that the results they obtained were accurate. These interviews were analysed to provide an overview of the techniques currently used when working with machine learning (ML) models. Opportunities for collaboration with human factors researchers to develop new tools are identified.
Jessica Baweja, Brett Jefferson, Corey Fallon
Open Access
Article
Conference Proceedings
Improving Trust in Power System Measurements
The power grid is a large and complex system. The system becomes larger and more complex daily. Distributed energy resources and a more active customer role are factors adding to the complexity. This complicated system is operated by a combination of human operators and automation. Effective control of the power grid requires an increasing amount of automation to support system operators. The need for more support from automation can only increase as operational complexity increases.Actions in controlling the system are entirely dependent on the results of measurements. Measurements inform decisions at all scales. How much trust can be placed in the measurements is essentially an unknown factor. North American Electric Reliability Corporation has generated reports showing that procedures and models have not always worked as expected. Part of the problem lies in the fact that system events can distort signal waveforms. Another part of the problem is that events taking place outside the control area of an operator can affect measured results. The companies involved, and their regulators, have had to change their requirements and guidelines.High “accuracy” measurements are available for most quantities of interest, but the problems are related to trustworthiness, rather than “accuracy.” Accuracy is established for a device within a controlled environment, where a “true value” can be estimated. Real-world conditions can be vastly different. The instrument may provide accurate output according to its specifications, but the measurement might not represent reality because what is happening in the real world is outside the bounds of these specifications. That is a problem that demands a solution. The crux of the matter is this: a real-world measurement’s usefulness as a decision-making aid is related to how believable the measurement is, and not to how accurate the owner’s manual says the instrument is. The concept of “uncertainty” that metrologists have refined over the last few decades is a statistical process that predicts the dispersion of future results. Such a measure is virtually meaningless for real-time power system use. The properties of the power system are not stationary for long periods. A low-quality result can lead to a bad decision, because power system measurements presently lack any kind of real-time “trustworthiness connection.”The signal model generally used in the electric power industry is that the voltages and currents are well-represented by mathematical sinusoids. Given that starting point, we describe two trust metrics that provide verifiable links to the real-time system being measured. The metrics capture any mismatch between the instrument measurement model and the actual signal. Our trust-awareness metrics can lead to ways to develop more robust operating models in the power system environment. Every measurement result is reported with an associated real-time trust (or no-trust) metric, allowing the user (whether human or not) to assess the usefulness of the result. It is, of course, up to the user to determine how a low-quality result should be used in decision-making. Examples of real-time trust metric calculations during real power system events are provided, with evaluation for application in utility user scenarios.
Artis Riepnieks, Harold Kirkham
Open Access
Article
Conference Proceedings
Can Machine Learning be a Good Teammate?
We hypothesize that successful human-machine learning teaming requires machine learning to be a good teammate. However, little is understood about what the important design factors are for creating technology that people perceive to be good teammates. In a recent survey study, data from over 1,100 users of commercially available smart technology rated characteristics of teammates. Results indicate that across several categories of technology, a good teammate must (1) be reliable, competent and communicative, (2) build human-like relationships with the user, (3) perform their own tasks, pick up the slack, and help when someone is overloaded, (4) learn to aid and support a user’s cognitive abilities, (5) offer polite explanations and be transparent in their behaviors, (6) have common, helpful goals, and (7) act in a predictable manner. Interestingly, but not surprisingly, the degree of importance given to these various characteristics varies by several individual differences in the participants, including their agreeableness, propensity to trust technology, and tendency to be an early technology adopter. In this paper, we explore the implications of these good teammate characteristics and individual differences in the design of machine learning algorithms and their user interfaces. Machine learners, particularly if coupled with interactive learning or adaptive interface design, may be able to tailor themselves or their interactions to align with what individual users perceive to be important characteristics. This has the potential to promote more reliance and common ground. While this sounds promising, it may also risk overreliance or misunderstanding between a system’s actual capabilities and the user’s perceived capabilities. We begin to lay out the possible design space considerations for building good machine learning teammates.
Leslie Blaha, Megan Morris
Open Access
Article
Conference Proceedings
Stress and Motivation on Reliance Decisions with Automation
The decision to rely on automation is crucial in high-stress environments where there is an element of uncertainty. It is equally vital in human-automation partnership that the human’s expectations of automation reliability are appropriately calibrated. Therefore, it is important to better understand reliance decisions with varying automation reliability. The current study examined the effects of stress and motivation on the decision to rely on autonomous partners. Participants were randomly assigned to a stress and motivation condition, using the Trier Social Stress Test (TSST) for stress induction, and monetary incentive for motivation. The main task was an iterative pattern learning task where one of two AI partners, one with high reliability and one with low reliability, gave advice at every iteration; the AI partner alternated every ten iterations. While motivation had a stronger effect than stress, both motivation and stress affected reliance decisions with the high reliability AI. The low reliability AI was affected to a lesser degree if at all. Overall, the decision to not rely on the AI partner, especially with the higher in reliability was slower than the decision to rely on the AI partner, with the slowest decision times occurring in the high stress condition with motivated participants, suggesting more deliberate processing was utilized when deciding against the advice of the AI higher in reliability.
Mollie Mcguire, Miroslav Bernkopf
Open Access
Article
Conference Proceedings
Human Factors for Machine Learning in Astronomy
In this work, we present a collection of human-centered pitfalls that can occur when using machine learning tools and techniques in modern astronomical research, and we recommend best practices in order to mitigate these pitfalls. Human concerns affect the adoption and evolution of machine learning (ML) techniques in both existing workflows and work cultures. We use current and future surveys such as ZTF and LSST, the data that they collect, and the techniques implemented to process that data as examples of these challenges and the potential application of these best practices, with the ultimate goal of maximizing the discovery potential of these surveys.
John Wenskovitch, Amruta Jaodand
Open Access
Article
Conference Proceedings
The History and Heritage of the Age of Simulation
Simulation of modern technologies has an important and informative history and an inspirational heritage. Simulation was utilised early in the development of aviation. Aircraft are controlled through a coordinated series of inputs from the pilot, similar to riding a horse. The difference is that falling from a horse is not as hazardous as falling from the sky. In response to this steep learning curve, the Antoinette simulator of 1910, operated by humans responding to the trainee´s inputs, was developed. World War I´s Allied and Central Powers utilised simulation to enhance combat effectiveness. Major Lanoe Hawker VC, of the Royal Flying Corp, pioneered British military simulators with a ´Rocking Fuselage´ for firing at a moving target, with a later version in which the ´Rocking Fuselage´ was mounted on a track. Hawker´s distinguished and innovative career abruptly ended when he was shot down and killed by Manfred von Richthofen. The advent of fly-by instruments and navigation by radio-directional beacons provided an ideal opportunity for enhanced simulation. Overcoming initial reluctance, a common historical occurrence with innovative technologies, Edwin Link combined his expertise and experience from the family´s piano and organ company to produce the iconic Link Trainer. The ability to incorporate communication from a ´ground controller´ and record on a map the pilot´s course enhanced the allies´ training programmes. The advent of shipboard radar, during WWII, in the maritime realm enabled operation in low or non-existent light situations, such as fog. However, this new technology resulted in a new class of accidents – misinterpretation of screen information leading to collisions. From the 1950s onwards, simulation has been integral to the training of deck officers in radar technology. In the late-1950s. N.S. Savannah, the United States´ atomic-powered merchant ship, pioneered civilian maritime simulation of a nuclear reactor and propulsion system. During the 1960s, maritime simulation was increasingly utilised to understand operation and crew performance better. In 1976, the use of CGI at the Computer Aided Operations Research Facility (CAORF), US Merchant Marine Academy, demonstrated the value of simulation in deck officer training. Increasingly, computers: analogue, electro-mechanical and digital, drove simulation forward. Early advances enhanced the experience for the operator and monitoring by the supervisor. DARPA´s pioneering role in the integration of ´networking, instrumentation and command and control´ has been transformative. This led to ´… outcomes that were in no way predictable, through after-the-fact were understandable.´ (Thorpe 2010)The material culture of simulation is in the collections of many museums – especially the Link Trainer. Most museum-based simulators are no longer operational due to malfunctions, lack of knowledge and concern about damage by "enthusiastic" public members. However, in a twist, there is interest in simulating simulators. The ´Rocking Fuselage inspires the WW1 Aviation Heritage Trust dogfight simulator´. In recent decades, the software associated with simulation has also gained its own historical archival value. Given the complexity of modern simulators and simulations, the question arises: what will be retained in museums and archives for future generations to engage with, personally or professionally, that records the Age of Simulation?
Bryan Lintott
Open Access
Article
Conference Proceedings
Human Factors in Discovery Phase of TRLs and HRLs
With rapid growth in technology, there has been a corresponding growth in research focused on the ways that human-machine interactions can be improved. As part of that work, researchers have explored how human expertise can inform technology design and evaluation. For example, interaction with subject matter experts (SMEs) or end users can help to design and enhance a machine. The human factors of technology release can be divided into five steps: discovery, planning, development, evaluation, and deployment. This framework is a higher-level abstraction of the Human Readiness Levels for technology use and adoption (See et al. 2018). In this exposition, we discuss how human factors methodologies, principles, and practices can be realized in the first phase, Discovery, of the technology development process.
Brett Jefferson, Jessica Baweja
Open Access
Article
Conference Proceedings
Assessing the Impact of Automated Document Classification Decisions on Human Decision-Making
As machine learning (ML) algorithms are incorporated into more high-consequence domains, it is important to understand their impact on human decision-making. This need becomes particularly apparent when the goal is to augment performance rather than replace a human analyst. The derivative classification (DC) document review process is an area that is ripe for the application of such ML algorithms. In this process, derivative classifiers (DCs), who are technical experts in specialized topic areas, make decisions about a document’s classification level and category by comparing the document with a classification guide. As the volume of documents to be reviewed continues to increase, and text analytics and other types of models become more accessible, it may be possible to incorporate automated classification suggestions to increase DC efficiency and accuracy. However, care must be taken to ensure that tool-generated suggestions do not introduce errors into the process, which could lead to disastrous impacts for national security. In the current study, we assess the impact of model-generated classification decisions on DC accuracy, response time, and confidence while reviewing document snippets in a controlled environment and compare them to DC performance in the absence of the tool (baseline). Across two assessments, we found that correct tool suggestions improved human accuracy relative to baseline, and decreased response times relative to baseline in one of these assessments. Incorrect tool suggestions produced a higher human error rate but did not impact response times. Interestingly, incorrect tool suggestions also resulted in higher confidence ratings when DCs made errors that aligned with the incorrect suggestion relative to cases in which they correctly disregarded its suggestion. These results highlight that while ML tools can enhance performance when the output is accurate, they also have the potential for impairing analyst decision-making performance if inaccurate. This has the potential for negative impacts on national security. Findings have implications for the incorporation of ML or other automated suggestions not only in the derivative classification domain, but also in other high-consequence domains that incorporate automated tools into a human decision-making process. The effects of factors such as tool accuracy, transparency, and DC expertise should all be taken into account when designing such systems to ensure the automated suggestions improve performance without introducing additional errors. SNL is managed and operated by NTESS under DOE NNSA contract DE-NA0003525
Mallory Stites, Breannan Howell, Phillip Baxley
Open Access
Article
Conference Proceedings