Human Factors in Robots, Drones and Unmanned Systems
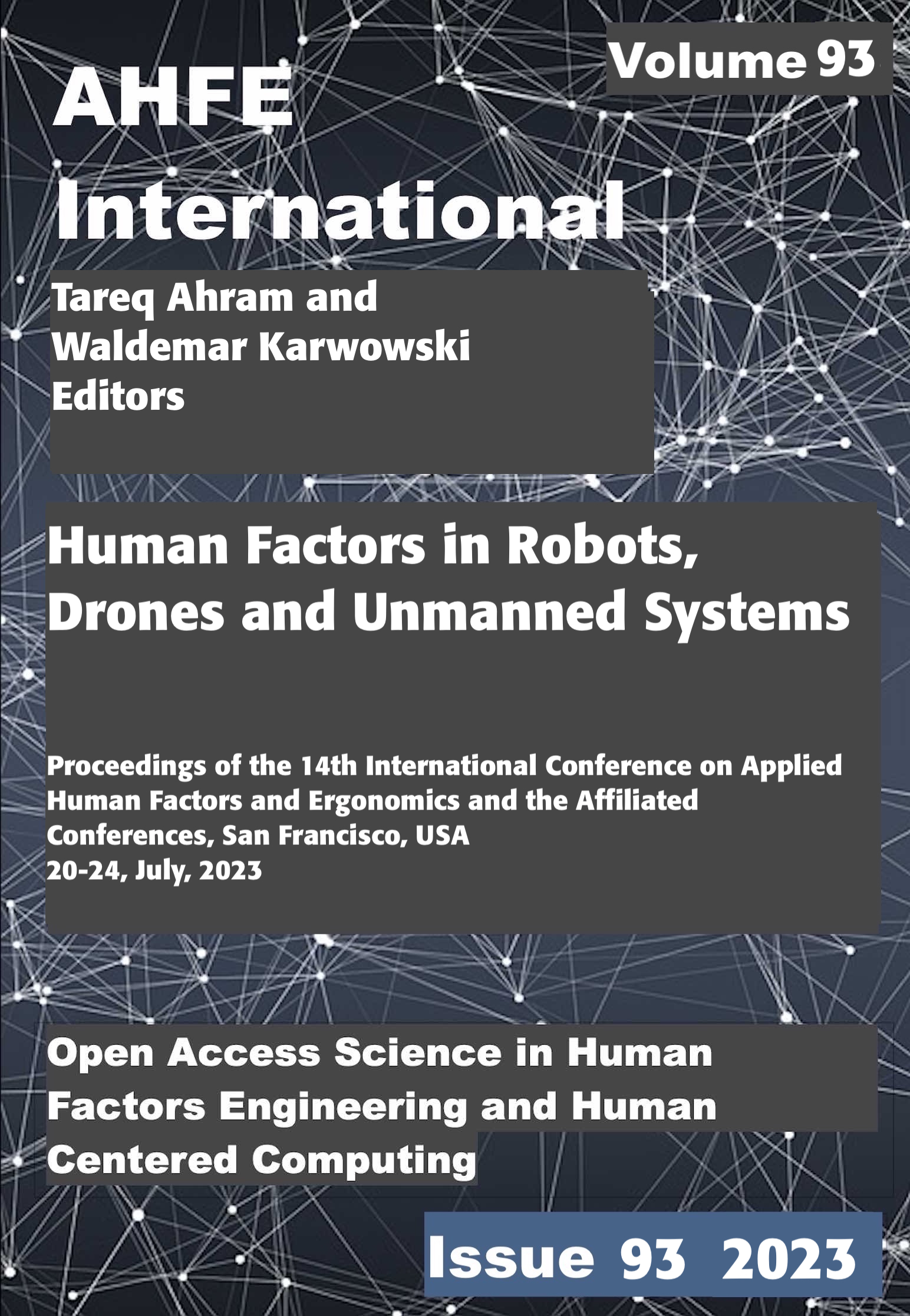
Editors: Tareq Ahram, Waldemar Karwowski
Topics: Robots, Drones and Unmanned Systems
Publication Date: 2023
ISBN: 978-1-958651-69-8
DOI: 10.54941/ahfe1003995
Articles
Navigating Team Cognition: Goal Terrain as Living Map to Situation Awareness
Deficits remain that preclude us from fully realizing effective teaming with autonomous systems, especially for hybrid teams of both humans and machine agents. One major impediment to agents assisting human users lies in the mutually intelligible communication of intent.How can we design agents to function in a more naturalistic way, with the ability to reason better about shared goals, and to perform necessary teamwork skills?To this end, we review the literature for teamwork models and team cognition in support of coordination and goal-centered reasoning. Then, we focus specifically on those processes and actions that enable bi-directional human machine communications for the development of shared situation awareness. From here, we develop an operational concept of Goal Terrain as an interaction mode that exists as both a reference product and as a method for communicating situation awareness in the form of events, status updates, and projections of goal-related outcomes. Goal Terrain is conceptualized as a schemata for representing team and individual goals to both human and machine agents, and it entails a graphic depiction of goals and subgoals, progress made against those goals, and projections about future feasibility and outcomes. It also includes a system for information sharing, to include notifications, alerts, and alarms intended to contextualize updated information to strengthen the development of mental models, highlight changes in the environment, and improve projections of outcomes. We expect that having a shared representation of the abstract tactical situation, shared goals, and associated risks to mission success will shorten communication time between agents. Framing events and updates in the context of objectives should improve team agility and responsiveness by allowing more tacit thought processes, intentions, and expectations to be communicated.Through the use of Goal Terrain, machine and human agents will be able to mutually support one another, create a greater sense of mutual predictability, and mutually adapt to dynamic needs and negotiate next steps. Human and machine agents alike will be able to proactively offer mutual assistance to one another by better monitoring individual and team activity and the relationships between subgoals and main goals. Agents will have a greater ability to offer insight and ask for guidance as appropriate, as they will have more information to work from regarding plan execution. Providing autonomous agents with enhanced context and a shared representation of goals and progress that they can query will reduce human interaction and intervention requirements. Agents with the ability to manipulate and represent goals in common with human agents should help to mitigate the problems of mixed-initiative interactions by managing the uncertainties of agent goals, focus of attention, plans, and status. In this paper, we construct a framework for how Goal Terrain might be utilized by different parties, human and agent alike, to accomplish shared tasks and outline how this might be tested to create a shared understanding in dynamic and uncertain environments.
Melissa Carraway, Victoria Chang, Nathan Hahn, Susan Campbell
Open Access
Article
Conference Proceedings
Third person drone – gamification motivated new approach for controlling UAV
Over the last years drones became a more and more popular solution for inspection and survey tasks. Controlling these drones, especially in tight spaces, using ‘line of sight’ or a ‘first person’ view from the perspective of the drone can be a difficult task. Often users experience an increased rate of difficulty that can be traced back to the limited situationaloverview of the user. To investigate whether a different form of visualization and interaction might result in a higher level of usability for the user, an experimental workspace was set up, with the goal of exploring the possibility of implementing a ‘third person view’ metaphor, like one used in video games. To further allow the user to experience his environment the use of virtual reality was used to stream the followers perspective directly to the users headset. This allowed the user to fly inside a simulated environment allowing for a control- and repeatable testing ground of the software. The workspace consisted of a simulation in which a ‘proof of concept’ was developed. In this simulation a drone used a conventional GPS sensor to follow a human controlled drone, offering his view, from a static camera, as a third person perspective to the controller using a virtual reality headset. Within the framework of the project, two aspects in particular were investigated: The performance of the technical system and the basic user experience and ergonomics of this form of interaction. To evaluate the performance of the follower system, the GPS position, as well as execution times and latencies were recorded. The user experience was evaluated based on personal interviews. The results show that the developed system can in fact follow a drone based on the GPS position alone, as well as calculate the desired positions in a timely manner. Yet, the existing delay in movement induced by the controller execution, as well as the drones own inertia did not allow for continues camera tracking of the drone using a static camera. This introduced several issues regarding tracking and impacted the user experience, but still showed that such a metaphor could in theory be implemented and further refined. The personal interviews showed that users would try tracking the drone by moving their head, like they are used to in virtual reality games. Ultimately, it was deduced that introducing a vectorbased drone movement, additional range detection sensor, as well as a moveable camera, controlled via head movement would be next steps to improve of the overall system. Since the prototype created in this paper only contained a bare bones user interface and experience the use of a usability study has been foregone in exchange for a more stable software solution. This allows further research into this topic the possibility of evaluating possible types of spatial user interfaces, which could improve the user immersion.
Thomas Hofmann, Dennis Linder, Philip Lensing
Open Access
Article
Conference Proceedings
Defining and Modeling AI Technical Fluency for Effective Human Machine Interaction
Working and interacting with artificial intelligence (AI) and autonomous systems is becoming an integral part of many jobs both in civilian and military settings. However, AI fluency skills, which we define as competencies that allow one to effectively evaluate and successfully work with AI, and the training that supports them have not kept pace with the development of AI technology. Specific subgroups of individuals who work in these areas, such as cyber and emerging technologies professions, are going to be required to team with increasingly sophisticated software and technological components, while also ensuring their skills are equivalent and not ‘overmatched’ by those of the AI. If not addressed, short term consequences of this gap may include degraded performance of sociotechnical systems using AI technologies and mismatches between humans’ trust in AI and the AI’s actual capabilities. In the long term, such gaps can lead to problems with appropriately steering, regulating, and auditing AI capabilities. We propose that assessing and supporting AI fluency is an integral part of promoting future appropriate use of AI and autonomous systems. The impact of AI fluency on the successful use of AI may differ depending on the role that the human has with the agent. For example, agents built on machine learning may update their behavior based on changes in the environment, changes in the task, or changes in input from humans. When an agent changes its behavior, humans must detect and adapt to the change. Furthermore, future agents may require human input to learn new behaviors or shape existing practices. These examples, where further intervention is needed from the human, emphasize the cyclic relationship between the agent and the human. However, humans vary in their ability to detect and respond to such changes based on their skills and experience. This example is just one potential aspect of the impact of one’s AI fluency on future human-AI interactions. To increment towards optimal performance, it is crucial to understand how and where differences in various aspects of AI fluency may help or hinder successful use of AI. The impact of AI fluency will be even stronger in the domain of interaction with autonomous systems built on AI technology, where agents may exhibit physical and information behaviors that affect human teammates’ safety. In this paper, we present a working definition and initial model of AI Technical Fluency (ATF) that relates predictors of ATF to potential outcome measures that would reflect one’s degree of ATF, including having accurate mental models of agents and the ability to interact with or use agents successfully. Additionally, we propose a preliminary set of assessments that might establish an individual’s ATF and discuss how (and the degree to which) different aspects of ATF may impact the various outcome measures. By gaining a better understanding of what factors contribute to one’s ATF and the impacts and limitations of ATF on the successful use of AI, we hope to contribute towards the ongoing research and development of new methods of interactions between humans and agents.
Susan Campbell, Rosalind Nguyen, Elizabeth Bonsignore, Breana Carter, Catherine Neubauer
Open Access
Article
Conference Proceedings
An Interactive Learning Framework for Item Ownership Relationship in Service Robots
Autonomous agents, including service robots, require adherence to moral values, legal regulations, and social norms to interact effectively with humans. A vital aspect of this is the acquisition of ownership relationships between humans and their carrying items, which leads to practical benefits and a deeper understanding of human social norms. The proposed framework enables the robots to learn item ownership relationships autonomously or through user interaction. The autonomous learning component is based on Human-Object Interaction (HOI) detection, through which the robot acquires knowledge of item ownership by recognizing correlations between human-object interactions. The interactive learning component allows for natural interaction between users and the robot, enabling users to demonstrate item ownership by presenting items to the robot. The learning process has been divided into four stages to address the challenges posed by changing item ownership in real-world scenarios. While many aspects of ownership relationship learning remain unexplored, this research aims to explore and design general approaches to item ownership learning in service robots concerning their applicability and robustness. In future work, we will evaluate the performance of the proposed framework through a case study.
Yuanda Hu, Yate Ge, Tianyue Yang, Xiaohua Sun
Open Access
Article
Conference Proceedings
A Bibliometric and Visual Analysis of autonomous vehicles-pedestrians interaction
In order to clarify the relevant concepts and research development process of the interaction between pedestrians and autonomous vehicles, this paper is based on the literature on the topic of "Interaction between pedestrians and autonomous vehicles" in the Web of Science database, with the help of visual knowledge graph analysis tools CiteSpace and VOSviewer. A knowledge map with keywords, countries and journals as nodes, and a knowledge map for interactive research between pedestrians and autonomous vehicles were built. This paper clarifies the research progress, research hotspots and development trends in the field of human-computer interaction between pedestrians and autonomous vehicles, and reveals the key research directions of human-machine interface (eHMI) outside the vehicle. Combining traditional literature review and bibliometric research methods, it provides Researchers and practitioners provide more detailed information on research progress in the field of human-vehicle interaction.
Chaomin Ma, Wanjia Zhang
Open Access
Article
Conference Proceedings
Human-agent teaming between soldiers and unmanned ground systems in a resupply scenario
Thanks to advances in embedded computing and robotics, intelligent Unmanned Ground Systems (UGS) are used more and more in our daily lives. Also in the military domain, the use of UGS is highly investigated for applications like force protection of military installations, surveillance, target acquisition, reconnaissance, handling of chemical, biological, radiological, nuclear (CBRN) threats, explosive ordnance disposal, etc. A pivotal research aspect for the integration of these military UGS in the standard operating procedures is the question of how to achieve a seamless collaboration between human and robotic agents in such high-stress and non-structured environments. Indeed, in these kind of operations, it is critical that the human-agent mutual understanding is flawless; hence, the focus on human factors and ergonomic design of the control interfaces.The objective of this paper is to focus on one key military application of UGS, more specifically logistics, and elaborate how efficient human-machine teaming can be achieved in such a scenario. While getting much less attention than other application areas, the domain of logistics is in fact one of the most important for any military operation, as it is an application area that is very well suited for robotic systems. Indeed, military troops are very often burdened by having to haul heavy gear across large distances, which is a problem UGS can solve.The significance of this paper is that it is based on more than two years of field research work on human + multi-agent UGS collaboration in realistic military operating conditions, performed within the scope of the European project iMUGS. In the framework of this project, not less than six large-scale field trial campaigns were organized across Europe. In each field trial campaign, soldiers and UGS had to work together to achieve a set of high-level mission goals that were distributed among them via a planning & scheduling mechanism. This paper will focus on the outcomes of the Belgian field trial, which concentrated on a resupply logistics mission.Within this paper, a description of the iMUGS test setup and operational scenarios is provided. The ergonomic design of the tactical planning system is elaborated, together with the high-level swarming and task scheduling methods that divide the work between robotic and human agents in the fieldThe resupply mission, as described in this paper, was executed in summer 2022 in Belgium by a mixed team of soldiers and UGS for an audience of around 200 people from defence actors from European member states. The results of this field trial were evaluated as highly positive, as all high-level requirements were obtained by the robotic fleet.
Geert De Cubber, Emile Le Flécher, Alexandre La Grappe - Dominicus, Daniela Doroftei
Open Access
Article
Conference Proceedings
Human factors assessment for drone operations: towards a virtual drone co-pilot
As the number of drone operations increases, so does the risk of incidents with these novel, yet sometimes dangerous unmanned systems. Research has shown that over 70% of drone incidents are caused by human error, so in order to reduce the risk of incidents, the human factors related to the operation of the drone should be studied. However, this is not a trivial exercise, because on the one hand, a realistic operational environment is required (in order to study the human behaviour in realistic conditions), while on the other hand a standardised environment is required, such that repeatable experiments can be set up in order to ensure statistical relevance. In order to remedy this, within the scope of the ALPHONSE project, a realistic simulation environment was developed that is specifically geared towards the evaluation of human factors for military drone operations. Within the ALPHONSE simulator, military (and other) drone pilots can perform missions in realistic operational conditions. At the same time, they are subjected to a range of factors that can influence operator performance. These constitute both person-induced factors like pressure to achieve the set goals in time or people talking to the pilot and environment-induced stress factors like changing weather conditions. During the flight operation, the ALPHONSE simulator continuously monitors over 65 flight parameters. After the flight, an overall performance score is calculated, based upon the achievement of the mission objectives. Throughout the ALPHONSE trials, a wide range of pilots has flown in the simulator, ranging from beginner to expert pilots. Using all the data recorded during these flights, three actions are performed:-An Artificial Intelligence (AI) - based classifier was trained to automatically recognize in real time ‘good’ and ‘bad’ flight behaviour. This allows for the development of a virtual co-pilot that can warn the pilot at any given moment when the pilot is starting to exhibit behaviour that is recognized by the classifier to correspond mostly to the behaviour of inexperienced pilots and not to the behaviour of good pilots.-An identification and ranking of the human factors and their impact on the flight performance, by linking the induced stress factors to the performance scores-An update of the training procedures to take into consideration the human factors that impact flight performance, such that newly trained pilots are better aware of these influences.The objective of this paper is to present the complete ALPHONSE simulator system for the evaluation of human factors for drone operations and present the results of the experiments with real military flight operators. The focus of the paper will be on the elaboration of the design choices for the development of the AI - based classifier for real-time flight performance evaluation.The proposed development is highly significant, as it presents a concrete and cost-effective methodology for developing a virtual co-pilot for drone pilots that can render drone operations safer. Indeed, while the initial training of the AI model requires considerable computing resources, the implementation of the classifier can be readily integrated in commodity flight controllers to provide real-time alerts when pilots are manifesting undesired flight behaviours.The paper will present results of tests with drone pilots from Belgian Defence and civilian Belgian Defence researchers that have flown within the ALPHONSE simulator. These pilots have first acted as data subjects to provide flight data to train the model and have later been used to validate the model. The validation shows that the virtual co-pilot achieves a very high accuracy and can in over 80% of the cases correctly identify ‘bad’ flight profiles in real-time.We propose the submission of this paper to the track “Human Factors in Robots, Drones and Unmanned Systems”
Daniela Doroftei, Geert De Cubber, Hans De Smet
Open Access
Article
Conference Proceedings
Ground Effect on a Landing Platform for an Unmanned Aerodynamic System
The ground effect is a phenomenon that takes place when an air vehicle is flying or hovering in vicinity of another surface, as this alters the airflow. Ground effect impacts inter alia flight stability which is a negative factor when landing. In this research we investigated a landing platform with a grid surface for a drone. 4 different textures for a landing platform were tested, a solid surface, a grid surface with hexagon cut-outs hovering in the air and the same grid with a solid surface 6cm below. We compared the vertical trust data of these to no surface within ground effect distance. The grid surface hovering in the air proved to have a 13% reduction of ground effect compared to the solid surface. While using the grid surface it is important to keep the distance of an underlying solid surface in mind. If the surface below the grid was too close, the positive effect was greatly reduced, making it no longer a preferable option to a solid surface. Therefore additionally, the minimal distance between the grid and the surface below was checked, for the second surface to be of no influence. This being 2 times the diameter of the rotor. This research shows potential for a grid surfaced landing platform, however due to stability issues while testing, further research on this topic is required.
Bruno Tardaguila, Stijn Claus, Manuel Martínez Herrero, Jolien De Wulf, Louis Nagels, Stijn Verwulgen
Open Access
Article
Conference Proceedings
Motion analysis of drone pilot operations and drone flight trajectories
This study compares the piloting practices and drone flight trajectories of skilled and novice drone pilots. Markers for 3D movement analysis were attached to the fingers that move the control stick. Similarly, drones were also marked and the flight movement of the drones analyzed. These two sets of data were cross-checked to examine the characteristics of the subjects. As a result, the following results were obtained.・The expert pilot did not adjust the position of the object directly in front of the object to be photographed, but at a distance of about 90 mm in the lateral direction.・The expert moved the drone in both the first axis and the second axis directions
Akihiko Goto, Naoki Sugiyama, Tomoko Ota
Open Access
Article
Conference Proceedings
Decision Transparency for enhanced human-machine collaboration for autonomous ships
Maritime Autonomous Surface Ships (MASS) are quickly emerging as a game-changing technology in various parts of the world. They can be used for a wide range of applications, including cargo transportation, oceanographic research and military operations. One of the main challenges associated with MASS is the need to build trust and confidence in the systems among end-users. While the use of AI and algorithms can lead to more efficient and effective decision-making, humans are often reticent to rely on systems that they do not fully understand. The lack of transparency and interpretability makes it very difficult for the human operator to know when an intervention is appropriate. This is why it is crucial that the decision-making process of MASS is transparent and easily interpretable for human operators and supervisors. In the emerging field of eXplainable AI (XAI), various techniques are developed and designed to help explain the predictions and decisions made by the AI system. How useful these techniques are in a real-world MASS operation is, however, currently an open question. This calls for research with a holistic approach that takes into account not only the technical aspects of MASS, but also the human factors that are involved in their operation. To address this challenge, this study employs a simulator-based approach were navigators test a mock-up system in a full mission navigation simulator. Enhanced decision support was presented on an Electronic Chart Display & Information System (ECDIS) together with information of the approaching ships as AIS (Automatic Identification System) symbols. The decision support provided by the system was a suggested sailing route with waypoints to either make a manoeuvre to avoid collision, or to maintain course and speed according to the Convention of the International Regulations for Preventing Collisions at Sea (COLREG). After completing the scenarios, the navigators were asked about the system's trustworthiness and interpretability. Further, we explored the needs for transparency and explainability. In addition, the navigators gave suggestions on how to improve the decision support based on the mentioned traits. The findings from the assessment can be used to develop a strategic plan for AI decision transparency. Such a plan would help building trust in MASS systems and improve human-machine collaboration in the maritime industry.
Andreas Madsen, Andreas Brandsæter, Magne V Aarset
Open Access
Article
Conference Proceedings
Mixed reality control of a mobile robot via ROS and digital twin
In recent years, mobile robotics has been increasingly used in agricultural production due to technological progress and increasingly powerful IT systems. Today, drones are already being used to spray agricultural areas with pesticides, for example. In the field of mobile robotics, control is currently based primarily on a controller or an app on a smartphone or tablet. If spatial target coordinates are to be specified, which the robot has to control, both systems quickly reach their limits. Using a mobile robot developed for the management of monocultures, this paper explains how spatial target coordinates can be approached with the help of a digital twin, ROS and a mixed reality interface using an MS HoloLens. The fusion of the technologies accordingly opens up new possibilities for a "human-robot interface".When developing an operating concept for the mobile robot, problems quickly arise regarding the determination of coordinates. The explanation for this is: The coordinate systems of the HoloLens and the mobile robot are not identical and, above all, are not known to the user. However, these obstacles can be eliminated by using a Digital Twin, which is displayed on the real robot using the HoloLens. By this measure, the coordinate system of the mobile robot is made known to the mixed reality device and it is possible to send the position coordinates as an offset to the current position of the robot. However, it should be noted that Unity (on the HoloLens) uses the left-hand rule, while ROS (on the robot) uses the right-hand rule. Therefore, the position data must be transformed accordingly.The same applies to the rotations, which must be converted as the equivalent of the coordinate transformation. Afterwards, the converted pose, which is now available in a "base_link" frame, can be transferred to the mobile robot via ROS.For the communication between the mixed reality device and the mobile robot, a ROS Bridge Client, which provides the messages in JSON format, is used. When sending target poses, it is important to note that the current time-stamped must be mandatory included in the message, otherwise the transformation from the "base_link" frame will not be converted to "map" coordinates. The transformation of the pose must also be done for the visual solution of the path planning. For the visualization, a distinction is made between the local and the global path planner A digital twin is superimposed on the real mobile robot for control and path planning. In the course of the project, problems with accuracy or performance have been discovered. In the intended application area - agriculture - the performance is weighted higher after an evaluation, so that slight position inaccuracies are accepted. The paper presents the concept as well as the interaction possibilities between the human and the mobile robot via the digital twin.
Carsten Wittenberg, Benedict Bauer, Nicholas Schloer
Open Access
Article
Conference Proceedings
Design and Acceptability of Technology: introduction to “Robotics & Design: the tool to design Human-Centered Assistive Robotics”
Assistive robotics is making significant progress in a wide variety of areas and will play a key role in the coming years as part of strategies for Ageing in Place and Active and Healthy Aging. Despite the demonstrated potential of technology to support the care of elderly and frail people, some elements still limit its application, such as the technology acceptability issue. The acceptability of technology, in particular for elderly and frail users, is a delicate issue, whose assessment metrics offer many opportunities for design research: in fact, the interaction that users establish with assistive technologies defines the very experience of aging (Forlizzi et al., 2004).The complexity of Human-Robot Interaction requires multidisciplinary collaboration that includes engineers, designers, health and social service associations and cooperatives, caregivers, economists, sociologists, lawyers, psychologists, therapists, and even end users such as the elderly and their families. In an effort to design for acceptability, it is therefore essential to make effective interdisciplinary cooperation among all professionals involved in the development of robotic systems. However, despite the common background in Human-Computer Interaction (HCI), the scientific and methodological approaches of Human-Robot Interaction (HRI) and Human-Centred Design (HCD) are significantly different in methods, philosophy and structure.The presented research is based on a general hypothesis: the HCD approach, if applied to the preliminary design phases of assistive robots, could lead to a deep understanding of needs, expectations and desires of people. Designers can use many methods (interviews, focus groups, ethnography, etc.) to explore people’s emotions and other abstract feelings that cannot be investigated through quantitative tools and statistical data. An appropriate knowledge of the user, of the context in which the interaction takes place and of the activities to be performed, could increase the attitude and intention of people to use assistive robots. This process would be even more effective if the designer knew the variables of acceptance in the HRI field. Designers often work within a multidisciplinary team composed of engineers, computer scientists, psychologists, sociologists, etc. Designers are catalysts for different professional skills involved in the project: consequently, they should also know the evaluation methods and intervention strategies in the field of HRI. This would lead designers to have a broader view on design processes and to recognize the most important variables of acceptability in robotics.On this basis, the tool "Robotics & Design: the tool to design Human-Centered Assistive Robotics," online at www.roboticsdesign.org, was developed. This tool, presented in this paper, has two main goals:- design purpose: to support the development of a cross-disciplinary collaborative process, to excerpt design patterns (Preece, 2015) from the results of scientific trials as they can be used by other designers according to users' features, activities, and contexts of use and then be translated into tangible design solutions;- theoretical and scientific purpose: to develop a methodological bridge between the HCD and HRI fields; and to provide designers and researchers in design with tools for agile consultation of the main methodologies and variables of acceptability in robotics and their intercorrelation.
Claudia Becchimanzi, Francesca Tosi
Open Access
Article
Conference Proceedings
Verification of a search-and-rescue drone with humans in the loop
In this presentation we use an example of a search-and-rescue drone, used by mountain rescue teams, to illustrate our approach to develop mathematical models and use them to verify behaviour that depends on human interactions with the drone. The design and development of human-in-the-loop robotic systems, such as the search-and-rescue drone, requires knowledge of the human, software, and hardware components of the system. The verification of these systems require knowledge of those same three components. Through this example we will demonstrate how a Hierarchical Task Analysis can be used to develop conformant sequence diagrams that can capture use cases of interest for verification. We will discuss the notation for our sequence diagrams, which is a variation of UML sequence diagrams tailored to capture time properties and with a view of the system that includes the software, the hardware, and human stakeholders.Our sequence diagram notation integrates within an existing verification framework, namely RoboStar, which provides domain-specific notations to model and verify both control software and robotic platforms. In the presentation, we show the kind of property and verification that we can carry out using our sequence diagrams and RoboStar technology. The presentation will also cover leading tools for modelling and verification of human-in-the-loop robotic systems, namely Circus, Ivy, PVSio-web and how they handle human behaviour within the system design. We will compare our approach with that supported by these tools.Verification is a technique used to prove that the system design and development meets the requirements specified; there are many forms of verification including formal verification, simulation, and testing. Formal verification is a tried and tested method to improve confidence in the correctness of a system, its ability to satisfy design requirements. Due to its application during design time, this confidence can be gained prior to the investing of time and resources into system development. Formal verification outputs mathematical proof artefacts that can be used in safety-case development. This verification technique requires formal models of system behaviour and formal models of the properties to be proved. To perform formal verification on a human-in-the-loop system, the formal model of the system behaviour needs to include a model of the expected human interaction. Whilst the data required for the generation of such a model can be provided by evidence from the fields of Human-Computer Interaction, Psychology, Human-Robot Interaction or Human Factors, this data needs to be gathered in a formal model for verification. On the other hand, requiring professionals with the knowledge of human behaviour to also have the expertise on formal verification is unrealistic. Our sequence diagrams are accessible and readable as a way to capture and communicate expected human behaviour. Moreover, it is possible to generate mathematical models for verification automatically from the sequence diagrams.
Holly Hendry, Mark Chattington, Ana Cavalcanti, Cade Mccall
Open Access
Article
Conference Proceedings
Human-Swarm Partnerships: A Systematic Review of Human Factors Literature
It is widely recognised that multiple autonomous agents operating together as part of a team, or swarm, could be used to assist in a variety of situations including search and rescue missions, warehouse operations and a number of military scenarios. From a sociotechnical perspective, these scenarios depict situations in which non-human and human agents are likely to work together in order to achieve a common goal. Unmanned Aerial Vehicles (UAVs) are often viewed as a convenient and cost effective way to gather information that is not easily accessible from any other means. However, we are beginning to see increasing efforts to scale up the autonomy of single-UAV systems to create aerial swarms. It is thought that aerial swarms may be used to assist in various situations including search and rescue missions, warehouse operations and military scenarios. Compared to a single robot, a swarm can provide a more efficient means to cover large areas and are scalable (i.e., can easily add or remove individual robots without significantly impacting the performance of the remaining group). Despite this, there has been some concern that Human Factors research into human-swarm partnerships is lacking. Thus, in order to understand the current ‘state of the art’, a systematic literature review was conducted to explore what Human Factors research is being conducted within the area of human-swarm partnerships and explore what design guidance exists to support the development of efficient and effective relationships. The initial search returned 143 articles. Duplicates were first removed and then the screening process involved filtering articles by titles then by abstract and then finally, full text. This approach led to 55 articles being retained. Inductive coding was used to identify themes within the text. This provided greater insight into the current focus of research with the context of human-swarm partnerships. A total of 5 themes were identified: interaction strategies, user interface design, management, operator monitoring and trust. However, the review also found that when it comes to design guidance, very little is available. One potential avenue for future research centre on the concepts of Meaningful Human Control and Effective Human Control. These concepts have been recognised as providing the foundation in which the design of human-swarm partnerships may be developed. This is because human agents are still likely to play a pivotal role in overall mission success and as such should retain full decisional awareness and possess a comprehensive understanding of the context of action in order for control to be meaningful. This implicates four of the research themes identified as part of this review: interaction strategies, user interface design, management and trust. Operator Monitoring, the final theme identified as part of this review, is indirectly linked to MHC and EHC because it acts as the mechanism in which operator engagement can be augmented. Arguably then, the building blocks to achieve MHC and EHC are beginning to take shape. However, more research is needed to bring this altogether in the quest for efficient and effective relationships between human agents and robot counterparts.
Victoria Steane, Jemma Oakes, Samson Palmer, Mark Chattington
Open Access
Article
Conference Proceedings
Using EAST to inform Systems Architecture Design: Considerations relating to the use of UAVs in Search and Rescue missions
There has been much interest in the use of Uncrewed Aerial Vehicles (UAVs) to support and extend missions within the Search and Rescue (SAR) space. However, detecting a human in the wilderness is a particularly challenging task. In the future, fitment of automated image classification aids may support UAV teams in correctly identifying targets within the environment thereby providing greater levels of support to ground search teams. The impact of such technology on the wider sociotechnical system however needs to be understood. This is because increasing the level of automation within a system can lead to degraded situation awareness, inappropriate calibration of trust and issues relating to complacency and technology overreliance. Within a SAR context, performance issues such as these could have disastrous consequences. In order to ensure systems are designed and integrated appropriately, it is essential that operator tasks are understood and that wider interactions are considered. This paper uses the Event Analysis of Systemic Teamwork (EAST) framework to sharpen the questions surrounding anticipated user and task requirements for UAV equipped SAR missions. A series of interviews with active members of Mountain Rescue teams across the United Kingdom were conducted using a condensed version of the Schema Action World (SAW) taxonomy. The subsequent analysis and network representations afforded by EAST appear to provide a platform in which the human view of the system can be investigated with a number of design recommendations proposed.
Victoria Steane, Sophie Hart, Jemma Oakes, Samson Palmer, Mark Chattington
Open Access
Article
Conference Proceedings
Community interface of gated communities as a docking space for future unmanned distribution
The research aims to integrate the system of unmanned logistics with community design to help communities cope with major public health crises. Through literature research and field investigation, it is found that the lack of community docking space and personnel is an important reason why unmanned logistics cannot be applied on a large scale at present. The study also observed that the last-mile delivery during the epidemic was physically hindered by the walled boundary of gated communities in China, which also made this type of space become a temporary transit place. It has the potential to become the touchpoint to integrate unmanned distribution and community space. This research uses the interface of the community as the transition medium for future unmanned distribution with the modular docking device attached to it. After the epidemic, such space can also be expanded into a diversified space with community social and entertainment attributes. Through the socialized development of logistics infrastructure, it can establish an interface-based logistics cooperation network to utilize the labor force active near the interface space and help the node connection of unmanned vehicles. In conclusion, this research can realize the diversification and socialization of unmanned logistics in the future, integrate distribution facilities into the community design through community interface, and promote the resilient development of community logistics to respond to the public health crisis.
Yanni Cai
Open Access
Article
Conference Proceedings
Autonomous human-machine teams: Data dependency and Artificial Intelligence (AI)
The reliance on concepts derived from observations in laboratories combined with the assumption that concepts and behavior are one-to-one (monism) have impeded the development of social science, machine learning (ML) and belief logics by restricting them to operate in controlled and stable contexts. Even in open contexts, using ideas developed in laboratories, despite using well-trained observers to make predictions about the likelihood of outcomes in open contexts, using the same concepts and assumptions, in 2016, Tetlock and Gardner's "superforecasters" failed to predict Brexit (Britain’s exit from the European Union) or Trump’s presidency. Similarly, in 2022, using traditional techniques, the CIA's expert observers and the Russian military planners both mis-judged the Ukranian people by claiming that Russia's army would easily defeat Ukraine. Providing support for overturning these concepts and assumptions, however, in 2021, the National Academy of Sciences made two claims with which we fully support. First, the Academy had warned that controlled contexts are insufficient to produce operational autonomous systems. We agree; by studying real-world contexts, we have concluded that the data derived from states of social interdependence not only create data dependency, but also that interdependence is the missing ingredient necessary for autonomy. Second, a team’s data dependency increases by reducing its internal degrees of freedom, thereby reducing its structural entropy production; this situation of heightened interdependence explains the Academy's second claim that the “performance of a team is not decomposable to, or an aggregation of, individual performances,” consequently providing corrobration for our new discipline of data dependency. We extend the Academy’s claims by asserting that the reduction of entropy production in a team’s structure (SEP), indicating the fittedness among team members, represents a tradeoff with a team’s performance, reflected by a team’s achievement of maximum entropy production (MEP).
William Lawless
Open Access
Article
Conference Proceedings
'Human-AI Teaming: Review of the NAS Report
Human-machine teams offer possibilities for conceptualization and action that could be achieved by neither alone. “Human-AI Teaming,” a recent report by the National Academies of Sciences observed that teams are not reducible to their aggregation: their individual performance does not entail successful team performance. The present paper selectively reviews the report and argues that their observation supports the development of a mathematical, behavioural, and physical model of human-machine teaming as a first, essential step toward integrating AI. Joint trade-offs between structural fitness and performance underlies such a model.
Ryan Quandt
Open Access
Article
Conference Proceedings
Leveraging Manifold Learning and Relationship Equity Management for Symbiotic Explainable Artificial Intelligence
Improvements in neural methods have led to the unprecedented adoption of AI in domains previously limited to human experts. As these technologies mature, especially in the area of neuro-symbolic intelligence, interest has increased in artificial cognitive capabilities that would allow an AI system to function less like an application and more like an interdependent teammate. In addition to improving language capabilities, next-generation AI systems need to support symbiotic, human-centered processes, including objective alignment, trust calibration, common ground, and the ability to build complex workflows that manage risks due to resources such as time, environmental constraints, and diverse computational settings from super computers to autonomous vehicles.In this paper we review current challenges in achieving Symbiotic Intelligence, and introduce novel capabilities in Artificial Executive Function we have developed towards solving these challenges. We present our work in the context of current literature on context-aware and self-aware computing and present basic building blocks of a novel, open-source, AI architecture for Symbiotic Intelligence. Our methods have been demonstrated effectively in both simulated crisis and during the pandemic. We argue our system meets the basic criteria outlined by DARPA and AFRL providing: (1) introspection via graph-based reasoning to establish expectations for both autonomous and team performance, to communicate expectations for interdependent co-performance, capability, an understanding of shared goals; (2) adaptivity through the use of automatic workflow generation using semantic labels to understand requirements, constraints, and expectations; (3) self healing capabilities using after-action review and co-training capabilities; (4) goal oriented reasoning via an awareness of machine, human, and team responsibilities and goals; (5) approximate, risk-aware, planning using a flexible workflow infrastructure with interchangeable units of computation capable of supporting both high fidelity, costly, reasoning suitable for traditional data centers, as well as in-the-field reasoning with highly performable surrogate models suitable for more constrained edge computing environments. Our framework provides unique symbiotic reasoning to support crisis response, allowing fast, flexible, analysis pipelines that can be responsive to changing resource and risk conditions in the field. We discuss the theory behind our methods, practical concerns, and our experimental results that provide evidence of their efficacy, especially in crisis decision making.
Eric Davis, Sourya Dey, Adam Karvonen, Ethan Lew, Donya Quick, Panchapakesan Shyamshankar, Ted Hille, Matt Lebeau
Open Access
Article
Conference Proceedings
AI Trust Framework and Maturity Model: Improving Metrics for Evaluating Security & Trust in Autonomous Human Machine Teams & Systems
The following article develops an AI Trust Framework and Maturity Model (AI-TFMM) to improve trust in AI technologies used by Autonomous Human Machine Teams & Systems (A-HMT-S). The framework establishes a methodology to improve quantification of trust in AI technologies. Key areas of exploration include security, privacy, explainability, transparency and other requirements for AI technologies to be ethical in their development and application. A maturity model framework approach to measuring trust is applied to improve gaps in quantifying trust and associated metrics of evaluation. Finding the right balance between performance, governance and ethics also raises several critical questions on AI technology and trust. Research examines methods needed to develop an AI-TFMM. Validation tests of the framework are run and analyzed against the popular AI technology (Chat GPT). OpenAI's GPT, which stands for "Generative Pre-training Transformer," is a deep learning language model that can generate human-like text by predicting the next word in a sequence based on a given prompt. ChatGPT is a version of GPT that is tailored for conversation and dialogue, and it has been trained on a dataset of human conversations to generate responses that are coherent and relevant to the context. The article concludes with results and conclusions from testing the AI Trust Framework and Maturity Model (AI-TFMM) applied to AI technology. Based on these findings, this paper highlights gaps that could be filled with future research to improve the accuracy, efficacy, application, and methodology of the AI-TFMM.
Michael Mylrea, Nikki Robinson
Open Access
Article
Conference Proceedings
Multiple Agents Interacting via Probability Flows on Factor Graphs
Expert team decision-making demonstrates that effective teams have shared goals, shared mental models to coordinate with minimal communication, establish trust through cross-training, and match task structures through planning. The key questions: Do best practices of human teams translate to hybrid human-AI agent teams, or autonomous agents alone? Is there a mathematical framework for studying shared goals and mental models? We propose factor graphs for studying multi-agent interaction and agile cooperative planning. One promising avenue for modeling interacting agents in real environments is with stochastic approaches, where probability distributions describe uncertainties and imperfect observations. Stochastic dynamic programming provides a framework for modeling multiple agents as scheduled and interacting Markov Decision Processes (MDPs), wherein each agent has partial information about other agents in the team. Each agent acts by accounting for both its objectives and anticipated behaviors of others, even implicitly. We have shown that Dynamic Programming, Maximum likelihood, Maximum entropy and Free-energy-based methods for stochastic control are special cases of probabilistic message propagation rules on modeled factor graphs. Now we show how multiple agents, modeled as multiple interacting factor graphs, exchange probability distributions carrying partial mutual knowledge. We demonstrate the ideas in contexts of moving agents on a discrete grid with obstacles and pre-defined semantic areas (grassy areas, pathways), where each subject has a different destination (goal). The scheduling of agents is fixed a priori or changes over time, and the forward-backward flow for each agent’s MDP is computed every time step, with additional branches that inject probability distributions into and from other agent MDPs. These interactions avoid collisions among agents and enable dynamic planning by agents, accounting for estimates of posterior probabilities of other agents states at future times, the precision and timing being adjustable. Simulations included limited interacting agents (three) on small rectangular discrete grid with starting points and destination goals, obstacles in various positions, narrow passages, small mazes, destinations that require coordination, etc. Solely due to probability distributions flowing in the interacting agent system, the solutions provided by the probabilistic model are interesting because agents that encounter potential conflicts in some regions autonomously adapt strategies, like waiting to let others pass, or taking different paths. The information available to each agent is a combination of rewards received from the environment and inferences about other agents. Previously, we described a scheme for a hierarchy (prioritized order) of agents and unique value function for each agent. Now, we propose a different, tunable interaction, wherein each agent dynamically transmits the posterior probability of its position at future time steps to other agents. The new framework allows flexibility in tuning the information that each agent has on others, ranging from complete knowledge of goals and positions about others out to a limited probabilistic awareness, both in precision and in time, for where others may be located at future time steps. This framework systematically addresses questions, such as the minimal amount of information needed for effective team coordination in the face of changes in goals, communication bandwidth, grid parameters and agent status.
Francesco Palmieri, Krishna Pattipati, Giovanni Di Gennaro, Amedeo Buonanno, Martina Merola
Open Access
Article
Conference Proceedings
Psychometric Properties of Team Resilience and Team Complementarity as Human-Autonomy Team Cohesion Factors
Adopting autonomous systems into human teams will likely affect the development of critical team states like cohesion. Thus, there is a need to understand how critical states emerge and change within human-autonomy teams and how they can be measured. To address these shortcomings, we developed a novel self-report scale to assess cohesion in human-autonomy teams. We created an initial pool of 134 items from the human team literature, selected to indicate the following dimensions: function-based task cohesion, structural cohesion (Griffith, 1988), interpersonal cohesion (Carron et al., 1985), and two novel subdimensions: perceived team complementarity (Piasentin & Chapman, 2007), and team resilience (Cato et al., 2018). Following assessment by eleven subject matter experts (SMEs), 82 items, were tested for content validity (Neubauer et al., 2021). We then administered items (or the scale) to participants during an online validation study. Although it is believed that all five subdimensions are useful for understanding cohesion in human autonomy teams, further analysis was warranted to evaluate the two new subdimensions. Therefore, the current paper focuses on the psychometric properties of team resilience and team complementarity.The online validation study was conducted at the U.S. Military Academy (USMA) at West Point using Qualtrics survey software. Data were collected from 294 USMA Cadets who ranged in age from 18 to 28 years (M= 19.97, SD= 1.49). We asked participants to imagine they were part of a human agent team that was instructed to work together. They viewed video vignettes illustrating these scenarios. These video clips featured high and low cohesive teams consisting of human and robot team members performing various collaborative tasks. Following the clip, participants rated their perceived level of the team’s cohesion using one or more subdimensions from our newly developed human-autonomy team cohesion scale. Participants also filled out a version of the Group Environment Questionnaire GEQ (Carless and DePaola, 2000).To evaluate our items and their corresponding subfactors, we defined several criteria for inclusion in subsequent research: internal consistency (i.e., whether different items measure the same underlying factor), invariance (i.e., whether items retain their meaning across contexts), sensitivity to depictions of high and low cohesion scenarios, and being both distinct from, and correlated with, the task and social cohesion subfactors from the GEQ-10. In our analyses of team complementarity, we found four items that met our inclusion criteria. In our analyses of team resilience, we first separated items into several subfactors: Team Learning Orientation, Shared Language, Team Functioning, and Perceived Efficacy (Berg et al., 2021; Morgan et al., 2013). Of the subfactors, only the Perceived Efficacy subfactor had good measurement properties. The Shared Language subfactor had good internal consistency and met criteria for partial scalar invariance, so it may contain helpful items in future measures. The results of these analyses highlight Team Complementarity as a salient subdimension for cohesion and suggest consideration for incorporating Perceived Efficacy into future Team Cohesion measurements.
Samantha Berg, Catherine Neubauer, Shan Lakhmani, Andrea Krausman, Sean Fitzhugh, Daniel Forster
Open Access
Article
Conference Proceedings
To Shoot or Not to Shoot? Human, Robot, & Automated Voice Directive Compliance with Target Acquisition & Engagement
The Army’s Optionally Manned Fighter Vehicle (OMFV) program seeks to, “...operate with no more than two crewmen”(Congressional Research Service, 2021), but currently uses four individuals: driver, gunner, commander, and ammo handler. This study sought to investigate how automated teammates affects warfighters within the tank. To achieve our research objective, we performed a human subjects’ study under IRB ID 5734, from the University of Virginia. This experiment was a mixed measures design as all participants were tasked to take directives from three entities, but half of the participants were given directives by a female voice while the other half were given a male voice from all entities. Participants were tasked to take commands from a human, NAO robot, and a computer automated voice while deciding on whether to fire upon armed robots, swarm of drones, or a single drone. They engaged targets by use of a computer mouse. Participants were instructed that the commands given to them might not be correct and it was upon their judgment if the target was indeed a necessary target. The entire experiment took approximately 30 minutes in total as there were 54 iterations where participants were given 20 seconds to respond with a click totaling 18 minutes that left them with 12 minutes where they completed a demographic survey, NASA TLX, SART, gave subjective feedback, and were briefed and debriefed. Data was analyzed using mixed linear model ANOVAs. Overall, army participants preferred instruction from a human. Less experienced users completely ignored all directives given and proceeded to engage as they saw fit. Individuals given directives from the computer had lower accuracy and situational awareness (SA) scores. Individuals directed by the computer had lower workload scores than they did being directed from a human, but higher workload scores than when directed by the robot. Human directed participants had a higher workload and situational awareness scores. Higher accuracy scores were seen in target acquisition, but not in target engagement for individuals directed by the robot. Participants receiving directives from the robot had the lowest workload score on average and had a moderate SA score. Participants never looked at the robot during the experiment once it began, as they were task saturated with their vision fixated on their targets while listening for commands. Participants felt the least workload from the robot but had moderate frustration with the robot and the highest frustration with the computer automated directives. There were significant differences found between the computer and robot directives when it came to SA (F(2,26) =3.48, p<.046, np2 = .211). There were also significant differences between the accuracy target engagement scores of the beginner and experienced participants (F(2,22) =3.83, p<.037, np2 = .258). There were no differences in how participants responded between male/female directives voices. Furthermore, the robot utilized did not show preference to male/female directives either to initiate mission directives. Ultimately, data produced in this study will help understand how to best facilitate operator performance with or without Human Automated Teammates.
Giovanna Camacho, Matthew Bolton, Joseph Loggi, Kallia Smith, Emmett Rice, Tariq Iqbal
Open Access
Article
Conference Proceedings